Welcome to DU!
The truly grassroots left-of-center political community where regular people, not algorithms, drive the discussions and set the standards.
Join the community:
Create a free account
Support DU (and get rid of ads!):
Become a Star Member
Latest Breaking News
Editorials & Other Articles
General Discussion
The DU Lounge
All Forums
Issue Forums
Culture Forums
Alliance Forums
Region Forums
Support Forums
Help & Search
Science
Related: About this forumAI models fed AI-generated data quickly spew nonsense
NEWS
24 July 2024
AI models fed AI-generated data quickly spew nonsense
Researchers gave successive versions of a large language model information produced by previous generations of the AI and observed rapid collapse.
By Elizabeth Gibney
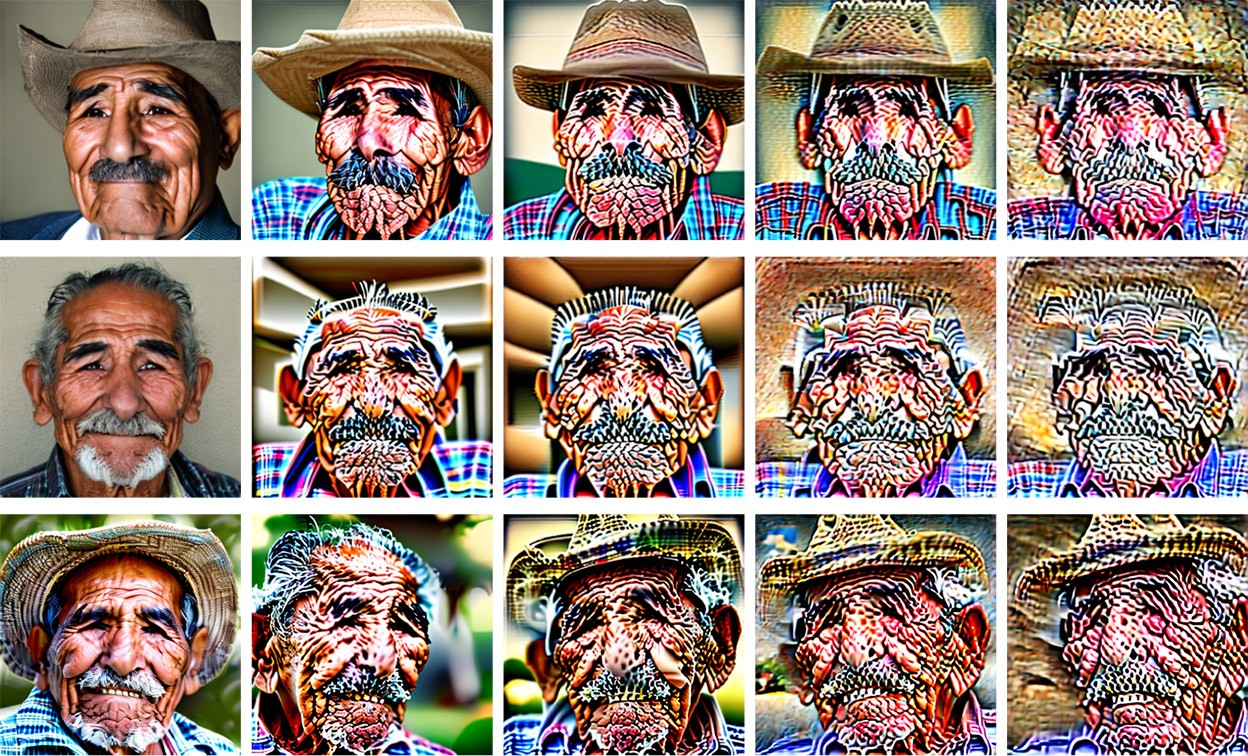
The increasingly distorted images produced by an artificial-intelligence model that is trained on data generated by a previous version of the model. Credit: M. Boháček & H. Farid/arXiv (CC BY 4.0)
Training artificial intelligence (AI) models on AI-generated text quickly leads to the models churning out nonsense, a study has found. This cannibalistic phenomenon, termed model collapse, could halt the improvement of large language models (LLMs) as they run out of human-derived training data and as increasing amounts of AI-generated text pervade the Internet.
The message is we have to be very careful about what ends up in our training data, says co-author Zakhar Shumaylov, an AI researcher at the University of Cambridge, UK. Otherwise things will always, provably, go wrong, he says. The team used a mathematical analysis to show that the problem of model collapse is likely to be universal, affecting all sizes of language model that use uncurated data, as well as simple image generators and other types of AI.
The researchers began by using an LLM to create Wikipedia-like entries, then trained new iterations of the model on text produced by its predecessor. As the AI-generated information known as synthetic data polluted the training set, the models outputs became gibberish. The ninth iteration of the model completed a Wikipedia-style article about English church towers with a treatise on the many colours of jackrabbit tails (see AI gibberish).
More subtly, the study, published in Nature1 on 24 July, showed that even before complete collapse, learning from AI-derived texts caused models to forget the information mentioned least frequently in their data sets as their outputs became more homogeneous.
[...]
24 July 2024
AI models fed AI-generated data quickly spew nonsense
Researchers gave successive versions of a large language model information produced by previous generations of the AI and observed rapid collapse.
By Elizabeth Gibney
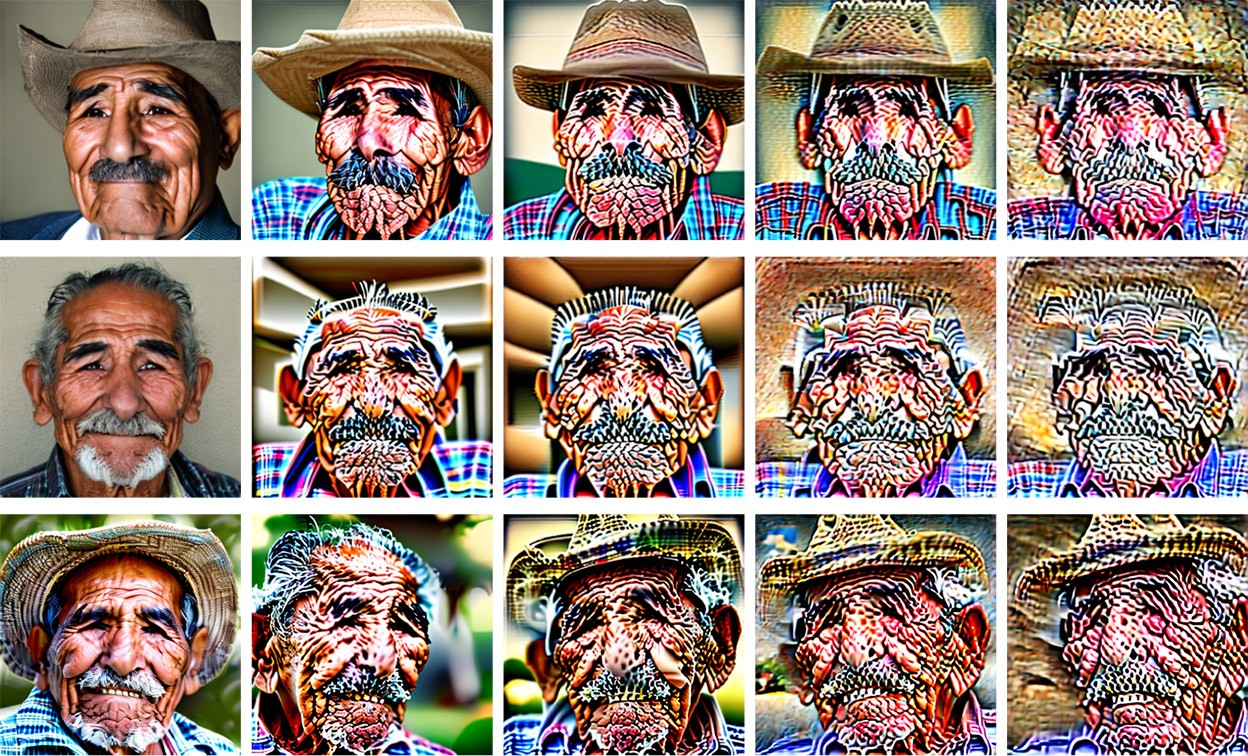
The increasingly distorted images produced by an artificial-intelligence model that is trained on data generated by a previous version of the model. Credit: M. Boháček & H. Farid/arXiv (CC BY 4.0)
Training artificial intelligence (AI) models on AI-generated text quickly leads to the models churning out nonsense, a study has found. This cannibalistic phenomenon, termed model collapse, could halt the improvement of large language models (LLMs) as they run out of human-derived training data and as increasing amounts of AI-generated text pervade the Internet.
The message is we have to be very careful about what ends up in our training data, says co-author Zakhar Shumaylov, an AI researcher at the University of Cambridge, UK. Otherwise things will always, provably, go wrong, he says. The team used a mathematical analysis to show that the problem of model collapse is likely to be universal, affecting all sizes of language model that use uncurated data, as well as simple image generators and other types of AI.
The researchers began by using an LLM to create Wikipedia-like entries, then trained new iterations of the model on text produced by its predecessor. As the AI-generated information known as synthetic data polluted the training set, the models outputs became gibberish. The ninth iteration of the model completed a Wikipedia-style article about English church towers with a treatise on the many colours of jackrabbit tails (see AI gibberish).
More subtly, the study, published in Nature1 on 24 July, showed that even before complete collapse, learning from AI-derived texts caused models to forget the information mentioned least frequently in their data sets as their outputs became more homogeneous.
[...]
=============================
https://www.nature.com/articles/s41586-024-07566-y
(full text, PDF, more, at link)
Article
Open access
Published: 24 July 2024
AI models collapse when trained on recursively generated data
Ilia Shumailov, Zakhar Shumaylov, Yiren Zhao, Nicolas Papernot, Ross Anderson & Yarin Gal
Nature volume 631, pages755759 (2024)Cite this article
Abstract
Stable diffusion revolutionized image creation from descriptive text. GPT-2 (ref. 1), GPT-3(.5) (ref. 2) and GPT-4 (ref. 3) demonstrated high performance across a variety of language tasks. ChatGPT introduced such language models to the public. It is now clear that generative artificial intelligence (AI) such as large language models (LLMs) is here to stay and will substantially change the ecosystem of online text and images. Here we consider what may happen to GPT-{n} once LLMs contribute much of the text found online. We find that indiscriminate use of model-generated content in training causes irreversible defects in the resulting models, in which tails of the original content distribution disappear. We refer to this effect as model collapse and show that it can occur in LLMs as well as in variational autoencoders (VAEs) and Gaussian mixture models (GMMs). We build theoretical intuition behind the phenomenon and portray its ubiquity among all learned generative models. We demonstrate that it must be taken seriously if we are to sustain the benefits of training from large-scale data scraped from the web. Indeed, the value of data collected about genuine human interactions with systems will be increasingly valuable in the presence of LLM-generated content in data crawled from the Internet.
Main
The development of LLMs is very involved and requires large quantities of training data. Yet, although current LLMs2,4,5,6, including GPT-3, were trained on predominantly human-generated text, this may change. If the training data of most future models are also scraped from the web, then they will inevitably train on data produced by their predecessors. In this paper, we investigate what happens when text produced by, for example, a version of GPT forms most of the training dataset of following models. What happens to GPT generations GPT-{n} as n increases? We discover that indiscriminately learning from data produced by other models causes model collapsea degenerative process whereby, over time, models forget the true underlying data distribution, even in the absence of a shift in the distribution over time. We give examples of model collapse for GMMs, VAEs and LLMs. We show that, over time, models start losing information about the true distribution, which first starts with tails disappearing, and learned behaviours converge over the generations to a point estimate with very small variance. Furthermore, we show that this process is inevitable, even for cases with almost ideal conditions for long-term learning, that is, no function estimation error. We also briefly mention two close concepts to model collapse from the existing literature: catastrophic forgetting arising in the framework of task-free continual learning7 and data poisoning8,9 maliciously leading to unintended behaviour. Neither is able to explain the phenomenon of model collapse fully, as the setting is fundamentally different, but they provide another perspective on the observed phenomenon and are discussed in more depth in the Supplementary Materials. Finally, we discuss the broader implications of model collapse. We note that access to the original data distribution is crucial: in learning tasks in which the tails of the underlying distribution matter, one needs access to real human-produced data. In other words, the use of LLMs at scale to publish content on the Internet will pollute the collection of data to train their successors: data about human interactions with LLMs will be increasingly valuable.
[...]
Open access
Published: 24 July 2024
AI models collapse when trained on recursively generated data
Ilia Shumailov, Zakhar Shumaylov, Yiren Zhao, Nicolas Papernot, Ross Anderson & Yarin Gal
Nature volume 631, pages755759 (2024)Cite this article
Abstract
Stable diffusion revolutionized image creation from descriptive text. GPT-2 (ref. 1), GPT-3(.5) (ref. 2) and GPT-4 (ref. 3) demonstrated high performance across a variety of language tasks. ChatGPT introduced such language models to the public. It is now clear that generative artificial intelligence (AI) such as large language models (LLMs) is here to stay and will substantially change the ecosystem of online text and images. Here we consider what may happen to GPT-{n} once LLMs contribute much of the text found online. We find that indiscriminate use of model-generated content in training causes irreversible defects in the resulting models, in which tails of the original content distribution disappear. We refer to this effect as model collapse and show that it can occur in LLMs as well as in variational autoencoders (VAEs) and Gaussian mixture models (GMMs). We build theoretical intuition behind the phenomenon and portray its ubiquity among all learned generative models. We demonstrate that it must be taken seriously if we are to sustain the benefits of training from large-scale data scraped from the web. Indeed, the value of data collected about genuine human interactions with systems will be increasingly valuable in the presence of LLM-generated content in data crawled from the Internet.
Main
The development of LLMs is very involved and requires large quantities of training data. Yet, although current LLMs2,4,5,6, including GPT-3, were trained on predominantly human-generated text, this may change. If the training data of most future models are also scraped from the web, then they will inevitably train on data produced by their predecessors. In this paper, we investigate what happens when text produced by, for example, a version of GPT forms most of the training dataset of following models. What happens to GPT generations GPT-{n} as n increases? We discover that indiscriminately learning from data produced by other models causes model collapsea degenerative process whereby, over time, models forget the true underlying data distribution, even in the absence of a shift in the distribution over time. We give examples of model collapse for GMMs, VAEs and LLMs. We show that, over time, models start losing information about the true distribution, which first starts with tails disappearing, and learned behaviours converge over the generations to a point estimate with very small variance. Furthermore, we show that this process is inevitable, even for cases with almost ideal conditions for long-term learning, that is, no function estimation error. We also briefly mention two close concepts to model collapse from the existing literature: catastrophic forgetting arising in the framework of task-free continual learning7 and data poisoning8,9 maliciously leading to unintended behaviour. Neither is able to explain the phenomenon of model collapse fully, as the setting is fundamentally different, but they provide another perspective on the observed phenomenon and are discussed in more depth in the Supplementary Materials. Finally, we discuss the broader implications of model collapse. We note that access to the original data distribution is crucial: in learning tasks in which the tails of the underlying distribution matter, one needs access to real human-produced data. In other words, the use of LLMs at scale to publish content on the Internet will pollute the collection of data to train their successors: data about human interactions with LLMs will be increasingly valuable.
[...]
4 replies
= new reply since forum marked as read
Highlight:
NoneDon't highlight anything
5 newestHighlight 5 most recent replies

AI models fed AI-generated data quickly spew nonsense (Original Post)
sl8
Jul 2024
OP
Very interesting. This is a weakness that will have to be solved before AI is fully viable in a lot of scenarios.
Martin68
Jul 2024
#3
It is asserted that "model collapse has not yet been seen in the 'wild' "
SorellaLaBefana
Jul 2024
#4
Think. Again.
(20,964 posts)1. Yep, once AI starts regurgitating it's own internet crap...
...it will only produce complete gibberish.
a term I first learned in 1968. Garbage In Garbage Out.
True in all computer processing of data... or human processing of data.
Martin68
(24,877 posts)3. Very interesting. This is a weakness that will have to be solved before AI is fully viable in a lot of scenarios.
SorellaLaBefana
(275 posts)4. It is asserted that "model collapse has not yet been seen in the 'wild' "
If it has not, then is simply another of those "when, not if" scenariossuch as the Climate Catastrophe
It is as though generations of academics were trained on Cliff's Notes which were based on papers written using only Cliff's Notes which had been newly abstracted from papers written in the same way